Innovative Data Science: Preparing for the Digital Age
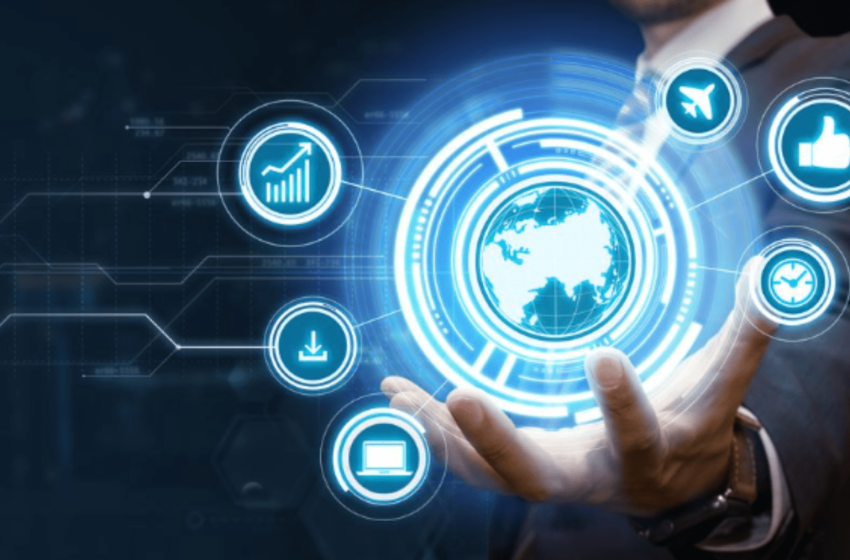
In today’s rapidly evolving digital landscape, data science stands as a cornerstone of innovation, reshaping industries, driving growth, and enabling unprecedented advancements. As businesses and individuals navigate the complexities of the digital age, understanding and leveraging data science has become essential. This article explores the transformative power of data science, highlighting its applications, challenges, and future prospects.
The Power of Data Science
In order to extract insights from data, the multidisciplinary area of data science integrates domain expertise, computer science, and statistics. It requires several steps, such as gathering, cleaning, analyzing, and interpreting data. Creating useful knowledge from raw data is the ultimate objective.
Applications Across Industries
Data science’s versatility allows it to be applied across numerous sectors, each reaping unique benefits.
-
Healthcare: In healthcare, data science improves patient outcomes by enabling personalized medicine. By analyzing large datasets of patient histories, genetic information, and treatment responses, data scientists can identify patterns that predict how patients will respond to specific treatments. This leads to more effective and individualized care plans.
-
Finance: The finance sector uses data science for risk management, fraud detection, and algorithmic trading. By analyzing historical financial data, data scientists develop models that predict market trends and identify fraudulent activities. This helps financial institutions make informed decisions and safeguard their assets.
-
Retail: Retailers leverage data science to enhance customer experience and optimize supply chains. By analyzing customer data, they can tailor marketing strategies to individual preferences, forecast demand, and manage inventory more efficiently. This not only boosts sales but also improves customer satisfaction.
-
Manufacturing: In manufacturing, data science helps in predictive maintenance and quality control. By monitoring equipment performance and analyzing production data, manufacturers can predict when machines are likely to fail and take proactive measures to prevent downtime. This increases efficiency and reduces costs.
Big Data and Machine Learning
Two key components of data science that have driven its recent advancements are big data and machine learning.
Big Data refers to the vast volumes of data generated every second by various sources such as social media, sensors, and transactions. The challenge lies not only in storing and managing this data but also in extracting meaningful insights from it. Advanced analytics and data processing tools enable data scientists to handle big data effectively.
Machine Learning As a branch of artificial intelligence, machine learning focuses on teaching algorithms to learn from data and make judgments or predictions without explicit programming. As machine learning models are exposed to additional data, they get better over time.. This capability is crucial for applications such as recommendation systems, autonomous vehicles, and natural language processing.
Challenges in Data Science
Despite its transformative potential, data science faces several challenges that need to be addressed to fully harness its capabilities.
Data Quality and Accessibility
One of the primary challenges is ensuring the quality and accessibility of data. Data of poor quality might produce imprecise models and deceptive findings. Data scientists spend a significant amount of time cleaning and preprocessing data to ensure its reliability. Additionally, data accessibility can be hindered by issues such as data silos and privacy concerns, making it difficult to obtain comprehensive datasets.
Skill Gap
There is a growing demand for skilled data scientists, but the supply has not kept pace. Data science requires a combination of technical skills, such as programming and statistical analysis, and domain-specific knowledge. Bridging this skill gap requires educational institutions to update their curricula and organizations to invest in training and development programs.
Ethical Considerations
As data science applications expand, ethical considerations become increasingly important. Issues such as data privacy, bias in algorithms, and the potential for misuse of data need to be addressed. Organizations must implement ethical guidelines and practices to ensure that data science is used responsibly and transparently.
Preparing for the Future
To thrive in the digital age, individuals and organizations must proactively prepare for the future of data science. Here are some strategies to consider:
Lifelong Learning
Given the rapid advancements in technology, continuous learning is essential. Professionals should stay updated with the latest trends and developments in data science by taking online courses, attending workshops, and participating in industry conferences. This ongoing education helps individuals remain competitive in the job market and ensures that they can leverage the latest tools and techniques.
Collaboration and Interdisciplinary Approach
Data science thrives on collaboration and an interdisciplinary approach. By working together, professionals from different fields can combine their expertise to solve complex problems. For example, data scientists can collaborate with healthcare professionals to develop predictive models for disease outbreaks or work with environmental scientists to analyze climate change data. Such collaborations lead to more comprehensive and impactful solutions.
Ethical Practices and Regulations
Organizations must prioritize ethical practices and comply with regulations when handling data. This includes implementing robust data governance frameworks, ensuring data privacy, and addressing algorithmic bias. By doing so, they can build trust with their customers and stakeholders and avoid potential legal and reputational risks.
Investing in Infrastructure
To fully exploit the potential of data science, organizations need to invest in the necessary infrastructure. This includes advanced data storage and processing systems, high-performance computing resources, and scalable analytics platforms. A robust infrastructure enables organizations to handle large datasets efficiently and derive valuable insights in real time.
The Future of Data Science
Looking ahead, the future of data science holds immense promise. Emerging technologies such as quantum computing, edge computing, and advanced machine learning algorithms will further expand the capabilities of data science. These innovations will enable faster and more accurate data processing, opening up new possibilities for applications across various sectors. To stay at the forefront of these advancements, professionals can benefit from the best Data Science Course in Noida, Delhi, Mumbai, Thane, Agra and all other cities in India. This knowledge will empower them to leverage new technologies effectively and drive innovation in their respective fields.
Quantum Computing
Quantum computing has the potential to revolutionize data science by solving complex problems that are currently beyond the reach of classical computers. Its ability to perform parallel computations at unprecedented speeds will enhance machine learning models, optimize logistics, and advance scientific research.
Edge Computing
Edge computing brings data processing closer to the source of data generation, reducing latency and improving efficiency. This is particularly important for applications such as autonomous vehicles and smart cities, where real-time data processing is crucial. By combining edge computing with data science, organizations can make faster and more informed decisions.
Advanced Machine Learning
Advancements in machine learning, including deep learning and reinforcement learning, will continue to push the boundaries of what is possible. These models will become more sophisticated, enabling applications such as natural language understanding, image recognition, and predictive analytics to reach new levels of accuracy and performance.
Conclusion
In the digital age, data science is a powerful tool that drives innovation and growth across industries. By understanding its applications, addressing its challenges, and preparing for its future, individuals and organizations can harness the full potential of data science. As we move forward, embracing data science will be crucial for staying competitive and thriving in an increasingly data-driven world. The journey of innovation is just beginning, and data science is at the forefront of this exciting transformation.